Design with Data-Driven Shape Rule Recognition through Learning-Based Shape Grammar Interpreter
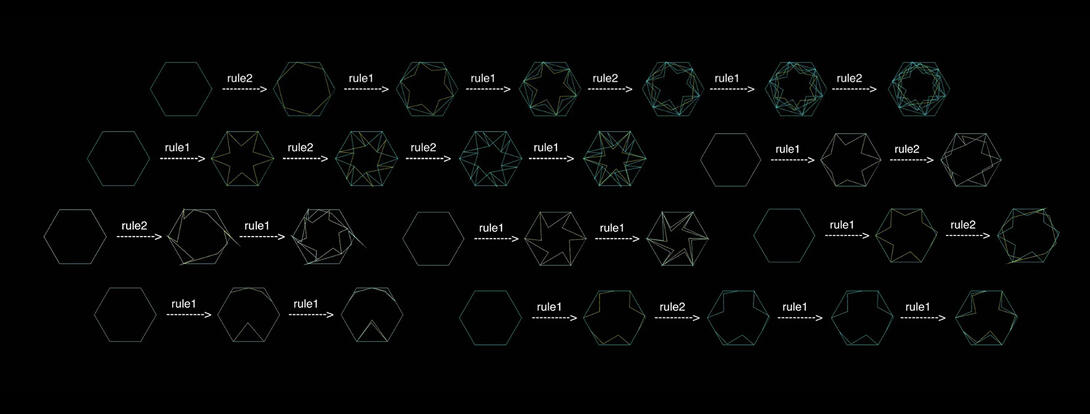
Advisors: Vernelle A. A. Noel, Daniel Cardoso Llach, Jinmo Rhee
Abstract: Shape grammar, which has been applied to design practices and research in various fields, is a rule-based system. Shape grammar interpreters still find it challenging to achieve computationally perfect sub-shape recognition in all dimension shapes and limit the generation of a variety of design alternatives because designers are required to generate shape rules based on their knowledge and expertise. However, with the rapid growth of artificial intelligence (AI) research, there have been increasing attempts to solve design problems using data-driven or learning-based methods through deep learning in architecture. Unlike the rule-based method, the learning-based method approaches the design problems by extracting the patterns of data inductively. How might a learning-based shape grammar interpreter, which helps designers recognize potential shape rules, affect the designer’s design approach, and change their design experience? The research approaches the questions by designing the learning-based shape grammar interpreter that infers rules and comparing it with the rule-based interpreters with simple geometry patterns through the user study. This study examines the changes in designers' design approaches and experiences and demonstrates the potential of data-driven methods to support rule-based systems. Such interaction with the interpreter may yield unexpected shape rules for designers and enrich the rule space.